ABSTRACT
With the escalation in
environmental concern and cost of production, researchers have focused on
investigating more efficient means of increasing grain yield while reducing
fertilizer use. This study was conducted to evaluate the use of normalized
difference vegetation index (NDVI) and derived coefficient of variation (CV)
to
predict grain
yield in corn (Zea
mays L.)
as effected by
hybrid, plant population, and
fertilizer N rate. A quadratic-plateau model existed between NDVI and plant
population, resulting in a critical NDVI, ranging from 0.74 to 0.78 in red NDVI
(RNDVI) and 0.65 to 0.66 in green NDVI (GNDVI). Conversely, the CV relationship
with plant population indicates that CV can recognize increased plant biomass at
levels well above the NDVI plateau and therefore can be utilized with NDVI to
determine plant biomass for yield prediction. The most effective growth stage
to predict grain yield was V8, presumably because the highest variability in
NDVI and CV occurred at this stage. Hybrid maturity did not effect grain yield
prediction at V8, but reproductive growth stage yield prediction may require
hybrid maturity categorization. The NDVI and CV determined using either the red
or green bands were equally effective for use in predicting corn grain yield
from early season sensor measurements.
As environmental concerns continue
to escalate and agricultural production becomes more scrutinized, new fertilizer
application practices will continue to be researched with the goal of increasing
fertilizer use efficiency. Currently, the Environmental Protection Agency (EPA)
is reporting that watersheds in all 48 states of the continental U.S.
tested for nitrate nitrogen (NO3-N) have groundwater
contamination levels above the maximum contaminant level (MCL), of which
Oklahoma is ranked 14th (EPA, 1999a). Production of cereal grains
has largely been held responsible for this groundwater contamination, in
particular corn (Zea mays L.) production, where high nitrogen (N) rates
have been applied in high yielding environments. Excessive N applications to
cereal grain crops continue to pollute the environment, increasing human health
risk and costing farmers needless additional expense along with negative
publicity. This exemplifies the need for continued research to improve
fertilizer use efficiency.
Raun and Johnson (1999) reported worldwide nitrogen use efficiency (NUE)
estimates to be approximately 33%, with developing countries estimated at 29%
and developed countries at 42%. As a result, N fertilizer losses were valued at
about $15.9 billion dollars annually, which as of August of 2001 has increased
to $20 billion dollars annually with the price of N fertilizer nearly doubling
due to the shortages of natural gas (Raun et al., 2002). There are several
paths in which N can be lost in the N cycle, dependent heavily on the crop and
environmental conditions surrounding it.
As split applications
of N became more popular, different methods have been used to measure early
season fertilizer use as a means of deciding how much more N fertilizer would be
needed to meet the predetermined grain yield goal. Several researchers looked
at in-season soil test of NO3-N at a depth of 30 cm, known
as presidedress NO3-N test (PSNT). Research from Vermont
showed that use of a PSNT reduced N rates without reducing grain yields in corn
by setting an N application limit with no N sidedress applications needed when
the PSNT is above 25 mg kg-1 NO3-N (Magdoff et
al., 1984; Durieux et al., 1995). While the PSNT worked well for the
northeastern USA, some adjustments were made to the PSNT for the semi-arid
regions under irrigation, particularly reducing the no N application limit to
about 15 mg kg-1 NO3-N (Spellman et al.,
1996). The PSNT test was further refined in corn production by Bundy and
Andraski (1995) when they reported that classifying soils into two categories
medium and high yield potential determined by: depth of root zone, water holding
capacity, and length of growing season, when the test values were considered in
the N responsive range. While the PSNT improved NUE, it could not produce these
results consistently, even when other soil properties were evaluated in tandem
with PSNT.
Chlorophyll meters (SPAD
meters) have been successfully used to determine in-season N status, since
chlorophyll content has been highly correlated with leaf N concentration (Wolfe
et al., 1988; Schepers et al., 1992). With the chlorophyll meters, researchers
developed an N Sufficiency index [(as-needed treatment/ well-fertilized
treatment) * 100] from which recommendations were made for in-season N
fertilizer applications when the index values fell below 95% (Blackmer and
Schepers, 1995; Varvel et al., 1997). Varvel et al. (1997) reported that
maximum grain yields in corn were attained when early season sufficiency indexes
ranged between 90 and 100% up to the V8 growth stage, but if the sufficiency
index fell below 90% at V8, maximum yields were not realized due to early season
N deficiency resulting in lost yield potential. Peterson et al. (1993)
indicated that variation in chlorophyll meter measurements can range up to 15%
from plant to plant, requiring considerable measurements in order to maintain a
representative average for the field at each sampling date. Nevertheless, the
chlorophyll meter was not a viable tool for guiding N side-dress decisions for
corn in the Southern Coastal Plain, undoubtedly because there was no
variable-rate application determination in the sufficiency index used, either
apply or dont apply (Gascho and Lee, 2002). Another drawback of the
chlorophyll meter is that by reading one leaf at a time, plant biomass cannot be
determined as with the remote sensor.
Using a
photodiode-based remote sensor measuring canopy radiance in the red (671nm) and
near-infrared (NIR, 780nm) spectral bands, Stone et al. (1996) developed a plant
N spectral index (PNSI) for correcting in-season wheat N deficiencies. This
index, the absolute value of the inverse of the normalized difference vegetation
index (NDVI), saved between 32 and 57 kg N ha 1 compared to fixed N
rates. Bausch and Duke (1996) developed an N Reflectance Index (NRI), using a
canopy reflectance ratio of NIR (760-900nm)/ green (520-600nm) for the low N
area to the NIR/ green for a well N-fertilized area, a very similar procedure to
the sufficiency index differing only in how the measurement is taken. Recent
work has shown that in-season normalized difference vegetation index {NDVI = [(NIRref/NIRinc)
(Redref/Redinc)]/[(NIRref/NIRinc)
+ (Redref/Redinc)]} highly correlated with final grain N
uptake (Lukina et al., 2001; Raun et al., 2002). Therefore, an in-season
response index (RI) from NDVI, referred to as RINDVI (Highest mean
NDVI N treatment/ Mean NDVI check treatment), was evaluated and determined to be
a viable method for measuring the potential response to additional N (Mullen et
al., 2003).
GopalaPillai and Tian
(1999) reported that by using linear regression models on normalized intensity
(NI) of a high-resolution color infrared (CIR) field image, they were able to
predict grain yield with 55 to 91% accuracy, depending on the field and growing
season. However, their work conceded that better correlation with yield was
obtained when using CIR images taken after pollination, than those from earlier
in the season, similar to the findings of work from Bartholome (1988) evaluating
better correlation to grain yield with accumulated NDVI after booting in millet
(Panicum miliaceum L.) and grain sorghum (Sorghum bicolor L.).
With the goal of improving correlation of NDVI to grain yield, Rasmussen (1998)
integrated the product of multitemporal NDVI with photosynthetically active
radiation, but was not successful since no single regression line was valid for
consecutive years. Other yield prediction models have been developed for corn,
although they do not use in-season plant health evaluations as part of the
equation. The crop environment resource synthesis (CERES)-Maize model simulates
the major physiological processes, soil water movement processes, and N
transformation and transport processes involved in plant growth and has been
studied a great deal and has been determined to simulate grain N uptake (Pang et
al., 1997). The artificial neural network (ANN) model was successful in
predicting grain yield, designed to evaluate the following three aspects of 15
input factors: (1) yield trends with temperature, rainfall, soil texture, and
soil pH, (2) interaction between N application and late July rainfall, and (3)
optimization of the input factors with a genetic algorithm (Liu et al., 2001).
However, both models use extensive evaluation of multiple factors to predict
final grain yield by full season simulation, but were not accurate for
mid-season grain prediction. As a
function of increasing NUE research, this study was conducted to evaluate
the potential of using NDVI to
determine N response and
predict grain yield in corn. The effects of
corn hybrid, plant population, and
fertilizer N rate on NDVI were also evaluated to establish what adaptations
might be necessary to use NDVI over a wide range of field conditions.
MATERIALS AND METHODS
Crop years 2002 and 2003
Two
experimental sites were established in the spring of 2002, one near Stillwater,
OK at the Lake Carl Blackwell Agronomy Research Farm (Pulaski fine sandy loam,
course-loamy, mixed, nonacid, thermic Typic Ustifluvent), and one near Haskell,
OK at the Eastern Oklahoma Research Station (Taloka silt loam fine, mixed,
thermic Mollic Albaqiustoll). Initial soil test results are reported in Table
1. The experiment employed a factorial arrangement within a randomized complete
block design with three replications. Individual plots measured 3.0 x 9.1 m
with 76.2 cm row spacing in conventional tillage. Ammonium nitrate (34-0-0) was
preplant surface-broadcasted by hand at rates of 0, 56, and 112 kg N ha-1.
Three Bacillus
thuringiensis (bt) gene enhanced corn hybrids identified by their
maturity date (105-day, 109-day, and 113-day) were planted at both sites in
2002, but three different hybrids without bt gene enhancement (104-day, 107-day,
111-day) were planted at both sites in 2003 (planting, fertilizer, and harvest
dates are reported on Table 2). Two different seeding rates were evaluated at
both sites: at the Haskell site seeding rates of 44,460 (low) and 66,690 seeds
ha-1 (high) in 2002 and 49,400 (low) and 71,630 seeds ha-1
(high) in 2003 and at the Lake Carl Blackwell (LCB) site, seeding rates of
35,568 (low) and 51,870 seeds ha-1 (high).
Each corn plot was
sensed with a GreenSeeker Hand Held optical reflectance sensor (Ntech
Industries, Ukiah, CA), measuring RNDVI {RNDVI = [(NIRref/NIRinc)
(Redref/Redinc)] / [(NIRref/NIRinc)
+ (Redref/Redinc)]}
and coefficient of variation (CV = standard deviation/mean) of the RNDVI
at different vegetative and reproductive growth stages at both sites each year
(sensing dates and growth stages with description presented in Table 3) with the
sensor nadir to the ground and approximately 70 cm above the crop canopy. Corn
grain was harvested with a Massey Ferguson 8XP experimental combine, removing 2
rows from the center of each plot. A Harvest Master yield-monitoring computer
installed on the combine was used to record grain weight and moisture levels.
Grain yield from each plot was determined by adjusting grain weight to 15.5%
moisture and a grain sub-sample was taken for total N analysis.
Crop year 2004
In the spring of 2004 the
experiment was reconfigured with the relocation of the Haskell trial to
an adjacent field at the same location with the same soil description.
Furthermore, plant populations were increased from two populations to four
(37050, 51870, 66690, and 81510 seeds ha-1) and corn hybrids were
reduced from three to two Bacillus thuringiensis (bt)
gene enhanced corn hybrids (99-day and 113-day). Initial soil test results and
planting, fertilizer, and harvest dates for the relocated Haskell site are also
reported in Tables 1 & 2. Individual plot size was reduced to 3.0 x 6.1 m with
the purpose of sustaining manageable labor requirements for the increased
treatment size. In addition, ammonium nitrate (34-0-0) application rates were
increased to 84 and 168 kg N ha-1 to assure N would not be a limiting
factor in high N application treatments.
With the
availability of a green NDVI
sensor, both GNDVI {GNDVI = [(NIRref/NIRinc) (Greenref/Greeninc)]
/ [(NIRref/NIRinc) + (Greenref/Greeninc)]}
and RNDVI were measured at different vegetative and reproductive growth
stages at both sites (sensing dates and growth stages are presented in Table 3)
with GreenSeeker Hand Held optical reflectance sensors. In addition, the CV
derived from both GNDVI (GCV) and RNDVI (RCV) was evaluated. The center two
rows of each corn plot were sensed separately with the sensor nadir to the
ground and approximately 70 cm above the crop canopy. Corn grain was harvested
(picked and shucked) by hand from the center two rows of each plot separately
and ear weights were recorded for each row. Four random ears from each row were
collectively weighed, dried in a forced air oven at 66oC, and weighed
again to determine moisture levels. Following the measurement of dry weights,
the four ears were shelled by hand using a Root-Healey
Manufacturing Company (Plymouth, OH) hand-crank corn sheller and the
grain weight was taken to determine an average cob weight for each row.
Finally, grain yield from each row was calculated by adjusting grain weight to
15.5% moisture and a grain sub-sample was taken for total N analysis.
Data analysis
Grain samples were dried in a forced air oven at 66oC, ground to pass
a 140 mesh sieve (100 um), and analyzed for total N content using a Carlo-Erba
NA 1500 automated dry combustion analyzer (Schepers et al., 1989). Nitrogen use
efficiency was determined using the difference method: dividing the difference
between the grain N uptake of the N treatment and the grain N uptake of the
check (0 N rate) by the N rate of the N treatment. Analyses of variance and
single degree of freedom contrasts were performed using SAS (SAS, 2002). Linear
and non-linear regression models were used to determine the relationships
present between grain yield and NDVI as well as between both NDVI and CV and the
treatment variables.
RESULTS AND DISCUSION
Plant Population
Crop Year 2002
Significantly higher RNDVI
and lower RCV values occurred in the higher plant population at both locations
for both the vegetative and reproductive sensor readings (data not shown).
Although plant biomass increased considerably between the V10 and R1 sensor
readings, RNDVI values decreased extensively due to tassel development at
Haskell. The lighter color of the tassel decreased red light absorbance in the
crop canopy. At both sites RCV declined between the first and second sensor
readings, indicating that an inverse relationship occurs between RNDVI and RCV,
as reflectance decreased from increased biomass absorbance, less variability was
detected.
Crop Year 2003
The high plant population
was significantly greater in RNDVI for all three hybrids at the first three
sensor readings (V6, V7, and V8) and at the V10 growth stage for the 104-day and
111-day hybrids. However, no differences were found within the plant
populations of any hybrid at the V16 growth stage. Although significant
treatment effects were visually present at V16, the effects could not be
measured effectively with RNDVI. Decreased RNDVI values were observed in the
high plant population of the 104-day hybrid at the R3 and R4 reproductive growth
stages as well as the R4 sensing of the 107-day hybrid. However, the 111-day
hybrid RNDVI increased in the higher plant population at the R1 and R2 growth
stages. While the 111-day hybrid may have obtained significantly higher RNDVI
values than either of the other two hybrids during the reproductive growth
stages, this occurred because of the maturity differences between the hybrids
(as the hybrid matures RNDVI decreased due to hastened lower leaf senescence)
since the 104-day and 107-day hybrids produced significantly higher grain
yields. The low plant population was significantly higher in RCV than the high
population for all hybrids at the V6, V7, and V8 growth stages. The low plant
population in the 104-day hybrid was higher in RCV than the high population at
V16 as well, but had a lower RCV at R4. The low plant population was higher in
RCV than the high population for both the 107-day and 111-day hybrids from R1 to
R3, but the high plant population was higher at R4 in the 107-day hybrid.
The high plant
population at the LCB site increased the RNDVI values and decreased the RCV
values of all three hybrids at all sensor (V6-R2) readings. At LCB, the decease
in RNDVI did not occur at tassel development, but this was essentially due to
the lower plant populations used. The greatest vegetative difference for both
RNDVI and RCV between the plant populations occurred around V8. The V8 sensor
data revealed that a quadratic-plateau relationship existed between measured
plant population and RNDVI for all three hybrids when combining both sites and
excluding measurements from the 0 N treatments (Figure 1). The 0N treatments
decreased RNDVI due to inadequate N, resulting in high variability in RNDVI that
overshadowed the variability associated with plant population. The power
function model best described the relationship between RCV and plant population
when combining both sites for all three hybrids (Figure 2).
Crop Year 2004
A positive linear response
to plant population in both GNDVI and RNDVI occurred for both hybrids at all
(V5-R5) sensor readings over both sites and negative linear responses to plant
population in GCV and RCV were seen at all sensor readings. The quadratic
response to plant population was variable between locations, hybrids, and NDVI
spectrums, however quadratic plateau models best described the relationship
between both GNDVI and RNDVI and plant population when the hybrids were
separately evaluated at the V8 growth stage (Figures 3 & 4). Power function
models best described the relationship between both GCV and RCV and Plant
population for both hybrids (Figures 5 & 6). The RNDVI relationship to plant
population was greater for both hybrids than the GNDVI relationship, although
the two NDVI spectrums had similar curves in both hybrids as was the case with
RCV compared to GCV.
As shown by the quadratic
plateau models (Figures 1, 3, & 4), the RNDVI plateau occurred in a narrow range
between 0.74 and 0.78, however the plant population at which the plateau
developed ranged greatly (between 49,000 & 62,000 plants ha-1)
reducing the accuracy of the combined model (Figure 7). This indicated that at
an RNDVI measurement of 0.76, increased biomass is no longer distinguishable,
while the plant population at which that occurs varies with hybrid and
environmental conditions. Similarly, the RCV relationship with plant population
was reduced in the combined model (Figure 8), however the power function
confirms that RCV can estimate plant biomass at RNDVI levels above the
plateau.
Nitrogen Response
Crop Year 2002
At Haskell a positive
linear response to N was seen for RNDVI in all three hybrids at the V10 reading,
but no N response was observed for RCV. While visual differences were observed
in the trial at the R1 reading, no significant trends were determined from
either RNDVI or RCV measurements and there was no linear response to N in grain
yield for any of the three hybrids at either plant population. Positive linear
responses to N were measured in RNDVI and RCV at LCB for all hybrids at both
readings, which was consistent with the grain yield data.
Crop Year 2003
A positive
linear RNDVI response to N occurred at Haskell in both plant populations of all
three hybrids at the V6, V7, and V8 sensor readings. As mentioned beforehand
canopy closure occurred at V16, at which time it was not possible to distinguish
observable N deficiencies (Figure 9). Negative linear RNDVI responses to N were
evaluated in the reproductive growth stages, particularly after the R1 growth
stage for both plant populations of all three hybrids at Haskell. Negative
linear RCV responses to N were observed at Haskell for V6, V7, V8, and R1 for
all hybrids, but positive linear RCV responses to N were observed at R3 and R4
for all hybrids (Figure 10). At tassel development RNDVI values declined,
however, the RNDVI values of the lower fertility treatments declined less since
tassel development was associated with plant health. Grain yield showed a
linear response to N in the 104-day hybrid, but not in either of the other two
hybrids. The lack of significant N response in the 107-day and 111-day hybrids
signifies late-season stress reduced grain fill and consequently the effects of
N deficiency since the earlier maturing 104-day hybrid was significantly higher
in grain yield. At LCB a positive linear RNDVI response to N occurred in the
low plant population of the 107-day hybrid for the R1 reading, no other RNDVI
responses to N were observed for either plant population of any hybrid and no N
responses were found in RCV. Grain yield data was consistent with RNDVI and RCV
measurements; no significant N response was found for any hybrid and/or plant
population at LCB.
Crop Year 2004
At Haskell
positive linear GNDVI and RNDVI responses to N were observed for both hybrids in
all plant populations during mid (V6-V9) and late (R4-R5) season sensor readings
(Figure 11). Negative linear GCV responses to N occurred for the 99-day hybrid
in the 66,690 and 81,510 plant populations at V8 and in the 66,690 at V9. In
the 113-day hybrid negative linear GCV responses were observed in the 37,050
plant population between V8 and V11 and in the 66,690 plant population at V8.
RCV was more sensitive to N response at Haskell with negative linear responses
for the 99-day hybrid in the 37,050 plant population at V5, V8, V9, and V11 and
in the 51,870 plant population at V5. Negative linear RCV responses in the
113-day hybrid occurred in the 37,050 plant population at all sensor readings
except V5 and in all four of the plant populations at V8 (Figure 12). Grain
yield showed a positive linear N response in the 37,050 plant population of both
hybrids and a quadratic N response in all of the higher plant populations of
both hybrids.
Positive linear GNDVI
responses to N observed at LCB were inconsistent within the 113-day hybrid with
sporadic responses occurring in the 37,050 and 66,690 plant populations and
consistent responses occurring in the 51,870 and 81,510 plant populations
between V7 and R4 growth stages. Positive linear GNDVI responses to N in the
99-day hybrid at LCB were not seen for the 37,050 population the entire season
or the other plant populations until the reproductive growth stages. Positive
linear RNDVI responses to N were limited to the 51,870 plant population of both
hybrids and the 81,510 of the 113-day hybrid between V7 and R4 growth stages.
Negative linear GCV responses to N were randomly observed in the reproductive
stages of the 113-day hybrid and no response in the 99-day hybrid. No responses
to N in RCV were observed for either hybrid as well. Grain yield data indicated
no N response in the 99-day hybrid and a positive linear response in the 81,510
plant population of the 113-day hybrid only. In general, the red band (RNDVI
and RCV) was more sensitive to N response than the green (GNDVI and GCV),
particularly in CV (Figures 11 & 12). Furthermore, the greatest variation
between the high N and 0 N treatments in both NDVI (GNDVI & RNDVI) and CV (GCV &
RCV) occurred approximately at the V8 growth stage, consistent with the RNDVI
and RCV results from 2003. Suggesting that the most accurate measurement of
biomass N response happens at V8, since high green reflectance and red
absorbance limits NDVI accuracy later in the vegetative stages.
Grain Yield Prediction
Crop Year 2002
The linear regression
model was evaluated between grain yield and RNDVI measurements and between grain
yield and RCV in 2002. Although the sensor measurements may have some
inaccuracy due to maintaining improper height above the crop canopy (i.e.
holding the sensor too close to the canopy), some very pronounced relationships
were found. Comparisons between grain yield and both RNDVI and RCV at early
growth stages (V7 at LCB, V10 at Haskell) resulted in poor relationships at both
sites (Tables 4 & 5), but comparisons made at the later reading (R1 growth
stage) showed a very good relationship between grain yield and both RNDVI and
RCV. The R1 sensor readings may have been more effective since tassel
development was affected by plant health and therefore narrowing the sensing
field of view by holding the sensor too close to the crop canopy would not
greatly affect the RNDVI measurement from a plant health prospective. At
earlier growth stages before tassel development, RNDVI measurements would need
to measure plant biomass which potentially could not be done effectively if the
sensor is held too close to the crop canopy, resulting in less variability
(lower RCV) between treatments and inflated RNDVI readings. Therefore
mid-season RNDVI can be utilized very effectively to predict grain yield.
Crop Year 2003
Linear regression
analysis revealed strong relationships between grain yield and RNDVI at early
growth stages (V6, V7, V8, and V10) in 2003 (Table 4). Similar results were
observed from linear regression between grain yield and RCV (Table 5). These
data indicate that early season grain yield prediction is achievable and
therefore sidedress N application based on grain yield prediction is practical.
Although lower than the V6 and V7 relationships with grain yield, the V8 growth
stage as previously mentioned showed the greatest variation between plant
populations and N rates, revealing that V8 growth stage sensor readings would
give the greatest indication of vegetative biomass growth whether or not it
translates to grain yield (Figures 13 & 14). The R2 growth stage comparison
between grain yield and RNDVI also showed a well-defined relationship supporting
the 2002 results that late season yield prediction is possible though not
practical for side-dress N application (Table 4). However, the R2 model showed
that each hybrid has a separate linear relationship with grain yield
corresponding to 2002 results and the model was improved significantly to that
of the V8 data when the hybrids were fitted with separate lines (data not
shown). Separating out the hybrids in the early season model did not improve
the relationship with grain yield, confirming that while significant differences
in RNDVI occurred between the hybrids at V8 these differences existed in grain
yield as well. Mid-season grain yield prediction can be achieved in the
reproductive stages as supported by the 2002 data, and in earlier vegetative
growth stages, particularly at V8 when the highest RNDVI variability occurred
between treatments.
Crop Year 2004
In 2004, the V8
data showed the highest positive linear relationship between both GNDVI and
RNDVI with grain yield (Table 4). However, the NDVI (both GNDVI & RNDVI) and CV
(both GCV & RCV) relationships with grain yield were considerably higher in the
99-day hybrid compared to the 113-day hybrid. Therefore developing separate
yield prediction lines for these two hybrids would have been necessary to
maintain accurate grain yield prediction. Little rationale can be given as to
why grain yield prediction using both NDVI and CV was improved for the 99-day
hybrid. Plant height differences between hybrids were small at V8, as early
maturing hybrids did not typically separate from the longer season hybrids in
this study (over 3 years) until the reproductive stages. The most plausible
explanation could be that the 99-day hybrid was not as well suited for the
dry-land environment as anticipated and was under substantial stress throughout
the growing season that limited yield potential early. Therefore, the 99-day
hybrid was potentially less sensitive to the post-sensing (late-season)
environment. While the red spectrum (RNDVI & RCV) had a distinct advantage over
the green (GNDVI & GCV) in relation with plant population and N response, there
were no advantages observed between the spectrums and grain yield (Tables 4 &
5). Poor relationships resulted from grain yield and the NDVI measurements
taken in the reproductive growth stages (R1-R5), possibly due to a violent
thunderstorm with damaging hail and strong winds that occurred between the V9
and V10 growth stages (June 2) that caused severe plant damage from torn leaves
and stunted plants due to lodging as proven by the lower RNDVI values at
V11-VT.
CONCLUSIONS
The critical
population at which the RNDVI plateau occurred ranged between 49,000 and 62,000
plants ha 1, however RNDVI plateau occurred in a narrow range
between 0.74 and 0.78. This indicated that increased biomass is no longer
distinguishable when RNDVI reaches 0.76, while the plant population at which
that occurs varies with hybrid and environmental conditions. The GNDVI resulted
in similar results in 2004, although the RNDVI had a distinct advantage over
GNDVI. Conversely, the RCV relationship with plant population indicated that
RCV can recognize increased plant biomass at levels well above the RNDVI plateau
and therefore can be utilized with RNDVI to determine plant biomass for yield
prediction.
The NDVI data from
the V8 growth stage predicted grain yield accurately in 2003 and 2004,
presumably because the highest variability in NDVI and CV occurred at the V8
growth stage both years. Later vegetative growth stages may actually contain
more plant variability than at V8 and could have stronger relationships with
grain yield. However canopy closure occurs shortly after V8 (V10 to V12) and
vegetative stage NDVI data collected thereafter cannot accurately account for
plant variability. Well-defined relationships between NDVI and grain yield also
occurred in the reproductive growth stages in 2002 and 2003, but at different
growth stages each year: R1 in 2001, R2 in 2003. Although late-season yield
prediction is not useful for N management and limited due to temporal
variability, the potential is there for other uses.
Separating the
hybrids improved these reproductive relationships with grain yield all three
years, but only improved the V8 relationship with grain yield in 2004. Hybrid
maturity did not effect grain yield prediction at V8, but reproductive growth
stage yield prediction will require hybrid maturity categorization. Finally,
comparisons made between the GNDVI and RNDVI relationships with grain yield in
2004 showed no significant differences over three locations.
REFERENCES
Bartholome, E. 1988.
Radiometric measurements and crop yield forecasting; Some observations over
millet and sorghum experimental plots in Mali. Int. J. Remote Sens.
9:1539-1552.
Bausch, W.C. and H.R.
Duke. 1996. Remote sensing of plant nitrogen status in corn. Trans. ASAE
39(5):1869-1875.
Blackmer, T.M. and J.S.
Schepers. 1995. Use of a chlorophyll meter to monitor nitrogen status and
schedule fertigation for corn. J. Prod. Agric. 8(1):56-60.
Bundy, L.G. and T.W.
Andraski. 1995. Soil yield potential effects on performance of soil nitrate
tests. J. Prod. Agric. 8:561-568.
Durieux, R.P., H.J. Brown,
E.J. Stewart, J.Q. Zhao, W.E. Jokela, and F.R. Magdoff. 1995. Implications of
nitrogen management strategies for nitrate leaching potential: Roles of nitrogen
source and fertilizer recommendations system. Agron. J. 87:884-887.
Gascho, G.J. and R.D. Lee.
2002. Determining side-dress nitrogen requirements of corn following broiler
litter in the Southern Coastal Plain. J. Plant Nutr. 11:2361-2371.
GopalaPillai, S. and L.
Tian. 1999. In-field variability detection and spatial yield modeling for corn
using digital aerial imaging. Trans. ASAE 42(6):1911-1920.
Liu, J., C.E. Goering, and
L. Tian. 2001. A neural network for setting target corn yields. Trans. ASAE
44(3):705-713.
Lukina, E.V., K.W. Freeman,
K.J. Wynn, W.E. Thomason, R.W. Mullen, A.R. Klatt, G.V. Johnson, R.L. Elliott,
M.L. Stone, J.B. Solie, and W.R. Raun. 2001. Nitrogen fertilization
optimization algorithm based on in-season estimates of yield and plant nitrogen
uptake. J. Plant Nutr. 24:885-898.
Magdoff, F.R., D. Ross, and
J. Amadon. 1984. A soil test for nitrogen availability to corn. Soil Sci.
Soc. Am. J. 48:1301-1304.
Menezes, R.S.C., G.J. Gascho,
W.W. Hanna, M.L. Cabrera, and J.E. Hook. 1997. Subsoil nitrate uptake by grain
pearl millet. Agron. J. 89:189-194.
Mullen, R.W., K.W. Freeman,
W.R. Raun, G.V. Johnson, M.L. Stone, and J.B. Solie. 2003. Identifying an
in-season response index and the potential to increase wheat yield with
nitrogen. Agron. J. 95:347-351.
Pang, X.P., J. Letey, and L.
Wu. 1997. Yield and nitrogen uptake prediction by CERES-Maize model under
semiarid conditions. Soil Sci. Soc. Am. J. 61:254-256.
Peterson, T.A., T.M.
Blackmer, D.D. Francis, and J.S. Schepers. 1993. Using a chlorophyll meter to
improve N management. NebGuide G93-1171-A. Cooperative Extension, Institute of
Agriculture and Natural Resources, Univ. of Nebraska-Lincoln.
Rasmussen, M.S. 1998.
Developing simple, operational, consistent NDVI-vegetation models by applying
environmental and climatic information. Part II: Crop yield assessment. Int.
J. Remote Sensing 19(1):119-139.
Raun, W.R., and G.V.
Johnson. 1999. Improving nitrogen use efficiency for cereal production. Agron.
J. 91:357-363.
Raun, W.R., J.B. Solie, G.V.
Johnson, M.L. Stone, R.W. Mullen, K.W. Freeman, W.E. Thomason, and E.V. Lukina.
2002. Improving nitrogen use efficiency in cereal grain production with optical
sensing and variable rate application. Agron. J. 94:815-820.
SAS Institute. 2002. The
SAS system for windows version 8.0. SAS Institute Inc., Cary, NC.
Schepers, J.S., D.D.
Francis, and M.T. Thompson. 1989. Simultaneous determination of total C, total
N, and 15N on soil and plant material. Commun. Soil Sci. Plant Anal.
20:949-959.
Schepers, J.S., D.D.
Francis, M. Vigil, and F.E. Below. 1992. Comparison of corn leaf nitrogen
concentration and chlorophyll meter readings. Commun. Soil Sci. Plant Anal.
23(17-20):2173-2187.
Spellman, D.E., A. Rongni,
D.G. Westfall, R.M. Waskom, and P.N. Soltanpour. 1996. Pre-sidedress nitrate
soil testing to manage nitrogen fertility in irrigated corn in a semi-arid
environment. Commun. Soil Sci. Plant Anal. 27:561-574.
Stone, M.L., J.B. Solie,
W.R. Raun, R.W. Whitney, S.L. Taylor, and J.D. Ringer.1996. Use of spectral
radiance for correcting in-season fertilizer nitrogen deficiencies in winter
wheat. Trans. ASAE. 39(5):1623-1631.
U.S. Environmental Protection
Agency (EPA). 1999a. National Primary Drinking Water Regulations Technical Fact
Sheets. Washington, D.C.: Office of Water, Office of Ground Water and Drinking
Water. http://www.epa.gov/OGWDW/hfacts.html. March
Varvel, G.E., J.S.
Schepers, and D.D. Francis. 1997. Ability for in-season correction of nitrogen
deficiency in corn using chlorophyll meters. Soil Sci. Soc. Am. J.
61:1233-1239.
Wolfe, D.W., D.W. Henderson,
T.C. Hsiao, and A. Alvino. 1988. Interactive
water and nitrogen effects on senescence of maize: II. Photosynthetic decline
and longevity of individual leaves. Agron. J. 80:865-870
Figure 1. Relationship between RNDVI and plant
population of three hybrids at the V8 growth stage over two locations with 0 N
treatments removed fitted to a quadratic-plateau model, 2003.
Figure 2. Relationship between RCV and plant
population of three hybrids at the V8 growth stage over two locations with 0 N
treatments removed, 2003.
Figure 3. Relationship
between plant population and both GNDVI and RNDVI at V8 with 0 N treatments
removed for the 99-day hybrid over two locations fitted to a quadratic-plateau
model, 2004.
Figure 4. Relationship between plant population
and both GNDVI and RNDVI at V8 with 0 N treatments removed for the 113-day
hybrid over two locations fitted to a quadratic-plateau model, 2004.
Figure 5. Relationship
between plant population and both GCV and RCV at V8 with 0 N treatments removed
for the 99-day hybrid over two locations, 2004.
Figure 6. Relationship between plant population
and both GCV and RCV at V8 with 0 N treatments removed for the 113-day hybrid
over two locations, 2004.
Figure 7. Relationship
between plant population and RNDVI at V8 with 0 N treatments removed for five
hybrids over four site-years fitted to a quadratic-plateau model.
Figure 8. Relationship
between plant population and RCV at V8 with 0 N treatments removed for five
hybrids over four site-years.
Figure 9. Effect of N rate
on RNDVI over time in the 104-day hybrid at the Haskell site, 2003.
Figure 10. Effect of N rate
on RCV over time in the 104-day hybrid at the Haskell site, 2003.
Figure 11. Effect of N rate
on both GNDVI and RNDVI over time in the 113-day hybrid at the Haskell site,
2004.
Figure 12. Effect of N rate
on both GCV and RCV over time in the 113-day hybrid at the Haskell site, 2004.
Figure 13. Relationship between grain yield and
RNDVI of the V8 growth stage over two locations, 2003. The 95% lower and upper
individual sample confidence intervals are represented by the bottom and top
lines, respectively.
Figure 14. Relationship between grain yield and
RCV of the V8 growth stage over two locations, 2003. The 95% lower and upper
individual sample confidence intervals are represented by the bottom and top
lines, respectively.
Table 1. Initial surface
(0-15cm) soil test results prior to experiment initiation at Haskell and Lake
Carl Blackwell (LCB), OK, 2002-04.
Sample |
NH4-N |
NO3-N |
P |
K |
pH |
------------------- mg kg-1
------------------- |
Haskell 2002-03 |
7.65 |
2.66 |
48 |
136 |
6.18 |
LCB 2002-04 |
14.28 |
1.63 |
23 |
239 |
6.13 |
Haskell 2004 |
NA |
20.00 |
112 |
218 |
6.70 |
NH4-N and NO3-N
2 M KCL extract; P and K Mehlich-3 extraction; pH 1:1 soil:deionized
water; NA- not available Haskell site relocated in 2004.
Table 2. Planting,
fertilizer, and harvest dates (dd-mm-yy) at Haskell and Lake Carl Blackwell (LCB),
OK, 2002-04.
Location |
Crop Year |
Planting |
Fertilizer Application |
Grain Harvest |
Haskell |
2002 |
18-4-2002 |
16-4-2002 |
11-9-2002 |
LCB |
2002 |
23-4-2002 |
23-4-2002 |
28-8-2002 |
Haskell |
2003 |
03-4-2003 |
03-4-2003 |
20-8-2003 |
LCB |
2003 |
01-4-2003 |
08-4-2003 |
07-8-2003 |
Haskell |
2004 |
01-4-2004 |
01-4-2004 |
31-8-2004 |
LCB |
2004 |
03-4-2004 |
03-4-2004 |
28-8-2004 |
Table 3. Sensing dates (dd-mm-yy
format) by growth stage at Haskell and Lake Carl
Blackwell (LCB), OK, 2002-04.
Growth
Stage |
Physiological Description |
--------
2002 ------- |
-------
2003 -------- |
-------
2004 -------- |
Haskell |
LCB |
Haskell |
LCB |
Haskell |
LCB |
V5 |
Ear and shoot development complete |
--- |
--- |
--- |
--- |
17-5-04 |
15-5-04 |
V6 |
Third whorl elongated, growing point
above surface |
--- |
--- |
22-5-03 |
21-5-03 |
22-5-04 |
18-5-04 |
V7 |
--- |
15-6-02 |
26-5-03 |
24-5-03 |
--- |
23-5-04 |
V8 |
Fourth whorl elongated, nutrient
deficiencies appear |
--- |
--- |
30-5-03 |
02-6-03 |
27-5-04 |
29-5-04 |
V9 |
--- |
--- |
--- |
07-6-03 |
31-5-04 |
01-6-04 |
V10 |
Rapid biomass growth, new leaves occur
every 2-3 days |
19-6-02 |
--- |
06-6-03 |
--- |
--- |
--- |
V11 |
--- |
--- |
--- |
--- |
08-6-04 |
--- |
V12 |
Brace roots develop, ear size and kernel
number determined |
--- |
--- |
--- |
--- |
--- |
08-6-04 |
V15-V16 |
--- |
--- |
15-6-03 |
14-6-03 |
--- |
--- |
VT
(Tasseling) |
Tassel development, maximum plant
height, pollen shed begins |
--- |
--- |
--- |
--- |
--- |
--- |
R1
(Silking) |
Silks develop and pollination occurs,
kernel fill begins |
11-7-02 |
09-7-02 |
21-6-03 |
20-6-03 |
15-6-04 |
16-6-04 |
R2
(Blister) |
Silks darken and dry, kernels white and
blister shaped, starch develops |
--- |
--- |
27-6-03 |
25-6-03 |
29-6-04 |
26-6-04 |
R3
(Milk) |
Kernels turn yellow externally, but
milking internal fluid |
--- |
--- |
07-7-03 |
--- |
--- |
--- |
R4
(Dough) |
Kernels thicken to a paste, 50% kernel
dry weight |
--- |
--- |
18-7-03 |
--- |
09-7-04 |
09-7-04 |
R5
(Dent) |
Kernels have dented at top, the milk
line appears |
--- |
--- |
--- |
--- |
16-7-04 |
17-7-04 |
Vegetative growth stages (V#) determined by
number of collared leaves. Depending on hybrid and environmental conditions
plants produce between 11 to 20 collared leaves (V11-V20), in 2004 only 12
collared leaves were observed before tasseling. --- No data available.
Table 4. Relationship between
grain yield and NDVI by growth stage and crop year fitted to a linear regression
model, 2002-04.
Coefficient of determination (R2) |
Growth Stage |
2002 |
2003 |
---------------------- 2004 ----------------------- |
All hybrids |
All hybrids |
99-day hybrid |
113-day hybrid |
RNDVI |
RNDVI |
GNDVI |
RNDVI |
GNDVI |
RNDVI |
V5 |
--- |
--- |
0.197 |
0.365 |
0.161 |
0.101 |
V6 |
--- |
0.781 |
0.381 |
0.332 |
0.145 |
0.113 |
V7 |
0.170 |
0.736 |
0.460 |
0.537 |
0.172 |
0.157 |
V8 |
--- |
0.622 |
0.577 |
0.605 |
0.423 |
0.412 |
V9 |
--- |
--- |
0.406 |
0.564 |
0.369 |
0.399 |
V10 |
0.164 |
0.650 |
--- |
--- |
--- |
--- |
V12 |
--- |
--- |
0.357 |
0.435 |
0.225 |
0.160 |
V15-V16 |
--- |
0.613 |
--- |
--- |
--- |
--- |
R1 |
0.859 |
0.086 |
0.210 |
0.335 |
0.366 |
0.254 |
R2 |
--- |
0.548 |
0.171 |
0.383 |
0.287 |
0.286 |
R3 |
--- |
0.192 |
--- |
--- |
--- |
--- |
R4 |
--- |
0.133 |
0.308 |
0.288 |
0.268 |
0.320 |
R5 |
--- |
--- |
0.285 |
0.334 |
0.362 |
0.364 |
Indicates only data from one site available. --- No data
available.
GNDVI = green normalized
difference vegetation index [(NIRref/NIRinc) (Greenref/Greeninc)]/[(NIRref/NIRinc)
+ (Greenref/Greeninc)]
NDVI = red normalized difference vegetation
index [(NIRref/NIRinc) (Redref/Redinc)]/[(NIRref/NIRinc) + (Redref/Redinc)]
Table 5. Relationship
between grain yield and CV by growth stage and crop year fitted to a linear
regression model, 2002-04.
Coefficient of determination
(R2) |
Growth Stage |
2002 |
2003 |
---------------------- 2004
----------------------- |
All hybrids |
All hybrids |
99-day hybrid |
113-day hybrid |
RCV |
RCV |
GCV |
RCV |
GCV |
RCV |
V5 |
--- |
--- |
0.023 |
0.211 |
0.082 |
0.096 |
V6 |
--- |
0.653 |
0.221 |
0.404 |
0.080 |
0.155 |
V7 |
0.172 |
0.719 |
0.243 |
0.394 |
0.060 |
0.105 |
V8 |
--- |
0.599 |
0.450 |
0.472 |
0.280 |
0.238 |
V9 |
--- |
--- |
0.392 |
0.407 |
0.187 |
0.220 |
V10 |
0.077 |
0.630 |
--- |
--- |
--- |
--- |
V12 |
--- |
--- |
0.429 |
0.361 |
0.128 |
0.117 |
V15-V16 |
--- |
0.475 |
--- |
--- |
--- |
--- |
R1 |
0.828 |
0.357 |
0.425 |
0.309 |
0.193 |
0.213 |
R2 |
--- |
0.483 |
0.234 |
0.328 |
0.223 |
0.185 |
R3 |
--- |
0.070 |
--- |
--- |
--- |
--- |
R4 |
--- |
0.144 |
0.227 |
0.224 |
0.203 |
0.177 |
R5 |
--- |
--- |
0.191 |
0.254 |
0.179 |
0.163 |
Indicates only data from
one site available. --- No data available.
GCV = green coefficient of
variation [standard deviation GNDVI/mean GNDVI]
RCV = red coefficient of
variation [standard deviation RNDVI/mean RNDVI]
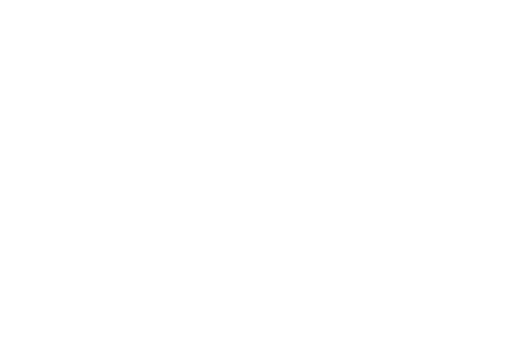
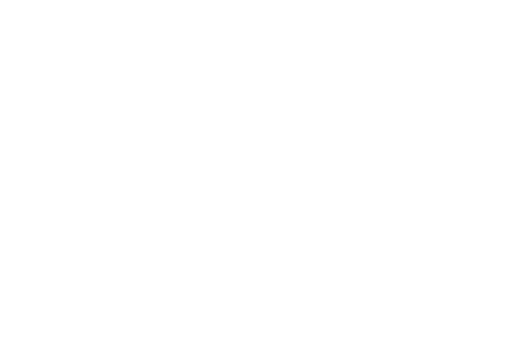
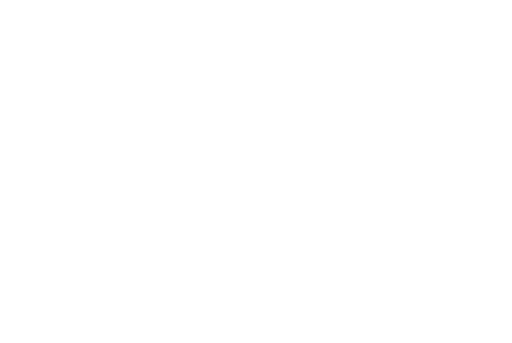
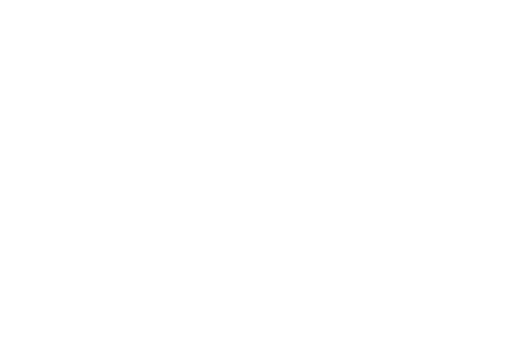
|